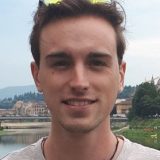
Olivier Petit
Semantic segmentation of 3D medical images with deep learning Deep Learning recently showed impressive results in visual recognition, especially with the performances given by convolutionnal neural networks (ConvNets) on the ImageNet challenge in 2012. Deep learning methods redefined the state of the art in all visual recognition challenges. Naturally, medical images analysis starts using these methods for all kind of tasks such as semantic segmentation. The main goal of this thesis is to research algorithms based on deep learning for semantic segmentation of 3D medical images, which consists of assigning a label (e.g. liver, stomach, pancreas, tumour, background) for every voxel in a volume. Setting up general methods which allow an automatic or semi-automatic deployment as well as the processing time (real-time) of a volume are key aspects of the project.
2022
Articles de revue
- 3D Spatial Priors for Semi-Supervised Organ Segmentation with Deep Convolutional Neural Networks. In International Journal of Computer Assisted Radiology and Surgery, 17 (1): 129-139, 2022. doi www
2021
Articles de revue
- Iterative Confidence Relabeling with Deep ConvNets for Organ Segmentation with Partial Labels. In Computerized Medical Imaging and Graphics: 101938, 2021. doi www
Articles de conférence
- U-Net Transformer: Self and Cross Attention for Medical Image Segmentation. In MICCAI workshop MLMI, Strasbourg (virtuel), France, 2021. www
2018
Articles de conférence
- Handling Missing Annotations for Semantic Segmentation with Deep ConvNets. In MICCAI workshop DLMIA, pages 20-28, Springer, Grenade, Spain, Lecture Notes in Computer Science book series (LNIP,volume 11045) , 2018. doi www